Starter Tutorial
This tutorial provides step-by-step instructions for getting started using EDSL (Expected Parrot Domain-Specific Language), an open-source Python library for simulating surveys, experiments and other research tasks using AI agents and large language models. EDSL is developed by Expected Parrot and available under the MIT License. The source code is hosted on GitHub.
Goals of this tutorial
We begin with technical setup: instructions for installing the EDSL library and storing API keys to access language models. Then we demonstrate some of the basic features of EDSL, with examples for constructing and running surveys with agents and models, and analyzing responses as datasets. By the end of this tutorial, you will be able to use EDSL to do each of the following:
Construct various types of questions tailored to your research objectives.
Combine questions into surveys and integrate logical rules to control the survey flow.
Design personas for AI agents to simulate responses to your surveys.
Choose and deploy large language models to generate responses for AI agents.
Analyze responses as datasets with built-in analytical tools.
Storing & sharing your work
We also introduce Coop: a platform for creating, storing and sharing AI-based research. Coop is fully integrated with EDSL and free to use. At the end of the tutorial we show how to use EDSL with Coop by posting content created in this tutorial for anyone to view at the web app. Learn more about how coop works in the EDSL documentation.
Note
You can also view and download the contents of this tutorial in a notebook at Coop
Further reading
In addition to this tutorial, please also see an Overview of features and common use cases for EDSL and a Survey logic checklist of tips for using EDSL effectively in the EDSL documentation page. To see recent research using or citing EDSL, see Papers & Citations in the documentation.
Questions
If you encounter any issues or have questions, please email us at info@expectedparrot.com or post a question at our Discord channel.
Pre-requisites
EDSL is compatible with Python 3.9 - 3.12. Before starting this tutorial, please ensure that you have a Python environment set up on your machine or in a cloud-based environment, such as Google Colab. You can find instructions for installing Python at the Python Software Foundation.
Recommendations
- Run code examples in a notebook
The code examples in this tutorial are designed to be run in a Jupyter notebook or another Python environment, or in a cloud-based environment such as Google Colab. If you are using Google Colab, please see additional instructions for setting up EDSL in the Colab setup page in the documentation.
- Use a virtual environment
We also recommend using a virtual environment when installing and using EDSL in order to avoid conflicts with other Python packages. You can find instructions for setting up a virtual environment at the Python Packaging Authority.
- Special instructions for Colab users
If you are using EDSL in a cloud-based environment, such as Google Colab, you can find additional instructions for setting up EDSL in the Colab setup page in the documentation.
Installation
To begin using EDSL, you first need to install the library. This can either be done locally on your machine or in a cloud-based environment, such as Google Colab. Once you have decided where to install EDSL, you can choose to whether install it from PyPI or GitHub:
- From PyPI
Install EDSL directly using pip, which is straightforward and recommended for most users. We also recommend using a virtual environment to manage your Python packages (see Recommendations above). Run the following command in your notebook to install EDSL from PyPI:
pip install edsl
- From GitHub
You can find the source code for EDSL and contribute to the project at GitHub. Installing from GitHub allows you to get the latest updates to EDSL before they are released to a new version at PyPI. This is recommended if you are using new features or contributing to the project. Run the following command to install EDSL from GitHub:
pip install git+https://github.com/expectedparrot/edsl.git@main
After installing EDSL, you can check the version that you have installed by running the following command in your notebook:
pip show edsl
To update your installation of EDSL to the latest version at PyPI, run the following command:
pip install --upgrade edsl
Accessing Language Models
The next step is to decide how you want to access language models. EDSL works with many popular language models that you can choose from to generate responses to your surveys. These models are hosted by various service providers, such as Anthropic, Azure, Bedrock, Deep Infra, DeepSeek, Google, Groq, Mistral, OpenAI, Replicate and Together. In order to run a survey, you need to provide API keys for the service providers of models that you want to use. There are two methods for providing API keys to EDSL:
Use an Expected Parrot API key to access all available models
Provide your own API keys from service providers
Managing keys
The easiest way to manage your keys is from your Expected Parrot account. Create an account with an email address and then navigate to your Keys page to find options for adding and sharing your keys:
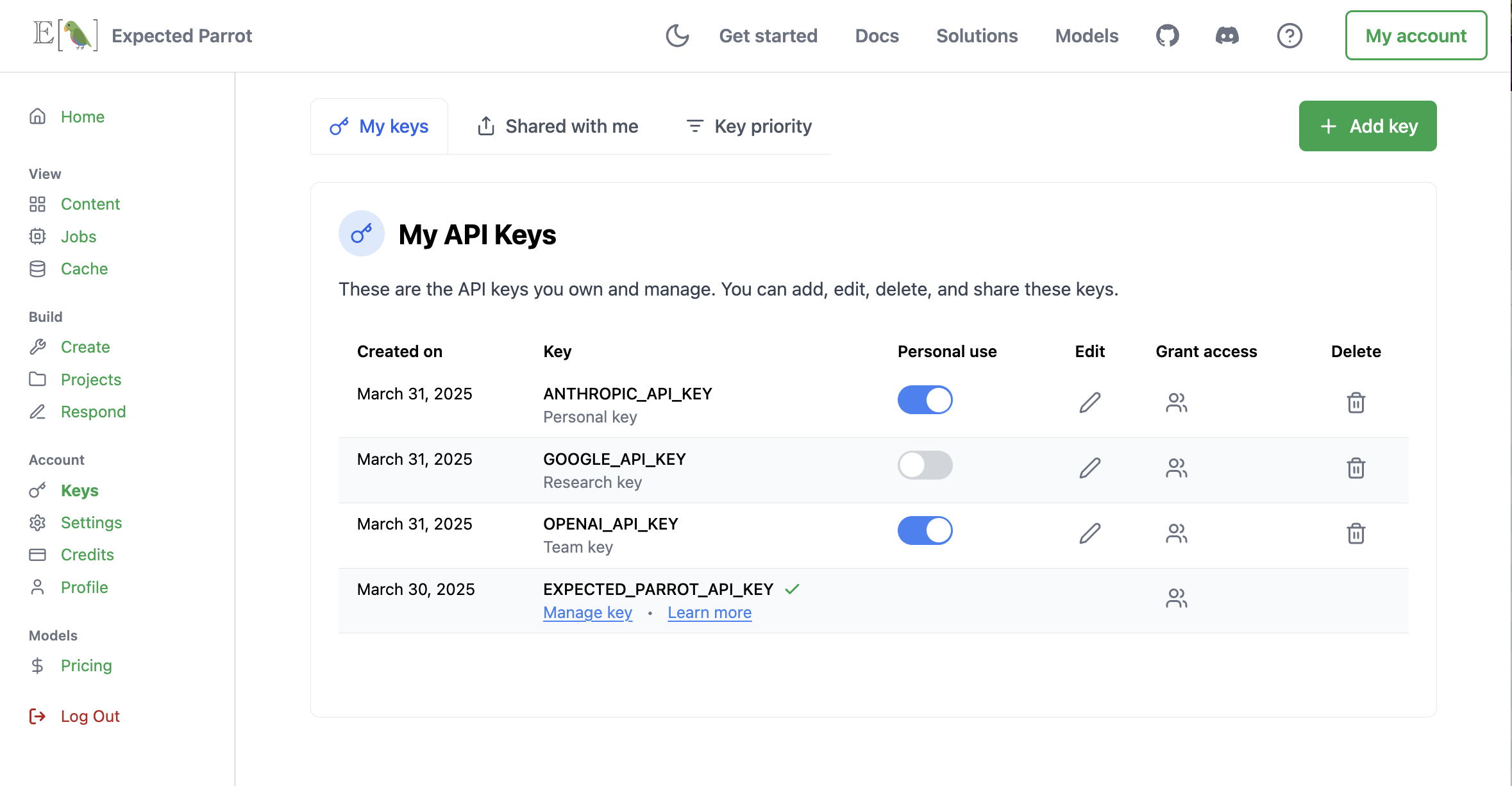
Your Expected Parrot key is automatically available to use by default whenever remote inference is activated. This key allows you to access the Expected Parrot server and run surveys with all available models. The key can be viewed at your Settings page where you can also find options for activating remote inference and caching. Activating these options allows you to run your surveys and store results at the Expected Parrot server instead of your own machine, using whichever keys you have prioritized.
Please see the Managing Keys section for more details on methods of storing and managing keys.
Note: If you try to run a survey without storing a required API key, you will be provided a link to activate remote inference and use your Expected Parrot key.
Credits & tokens
Running surveys with language models requires tokens. If you are using your own API keys, service providers will bill you directly. If you are using your Expected Parrot API key to access models, you will need to purchase credits to cover token costs. Please see the model pricing page for details on available models and their current prices.
Note: Your account comes with 2,500 free credits ($25 worth of API calls). You can purchase more credits at any time at your Credits page.
After installing EDSL and storing API keys you are ready to run some examples!
Example: Running a simple question
EDSL comes with a variety of question types that we can choose from based on the form of the response that we want to get back from a model. We can see a list of all question types and examples of each of them by running the following code:
from edsl import Question
Question.available()
Output:
question_type |
question_class |
example_question |
---|---|---|
checkbox |
QuestionCheckBox |
Question(‘checkbox’, question_name=”””never_eat”””, question_text=”””Which of the following foods would you eat if you had to?”””, min_selections=2, max_selections=5, question_options=[‘soggy meatpie’, ‘rare snails’, ‘mouldy bread’, ‘panda milk custard’, ‘McDonalds’], include_comment=False, use_code=True) |
extract |
QuestionExtract |
Question(‘extract’, question_name=”””extract_name”””, question_text=”””My name is Moby Dick. I have a PhD in astrology, but I’m actually a truck driver”””, answer_template={‘name’: ‘John Doe’, ‘profession’: ‘Carpenter’}) |
free_text |
QuestionFreeText |
Question(‘free_text’, question_name=”””how_are_you”””, question_text=”””How are you?”””) |
functional |
QuestionFunctional |
Question(‘functional’, question_name=”””sum_and_multiply”””, question_text=”””Calculate the sum of the list and multiply it by the agent trait multiplier.”””) |
likert_five |
QuestionLikertFive |
Question(‘likert_five’, question_name=”””happy_raining”””, question_text=”””I’m only happy when it rains.”””, question_options=[‘Strongly disagree’, ‘Disagree’, ‘Neutral’, ‘Agree’, ‘Strongly agree’]) |
linear_scale |
QuestionLinearScale |
Question(‘linear_scale’, question_name=”””ice_cream”””, question_text=”””How much do you like ice cream?”””, question_options=[1, 2, 3, 4, 5], option_labels={1: ‘I hate it’, 5: ‘I love it’}) |
list |
QuestionList |
Question(‘list’, question_name=”””list_of_foods”””, question_text=”””What are your favorite foods?”””, max_list_items=None) |
matrix |
QuestionMatrix |
Question(‘matrix’, question_name=”””child_happiness”””, question_text=”””How happy would you be with different numbers of children?”””, question_items=[‘No children’, ‘1 child’, ‘2 children’, ‘3 or more children’], question_options=[1, 2, 3, 4, 5], option_labels={1: ‘Very sad’, 3: ‘Neutral’, 5: ‘Extremely happy’}) |
multiple_choice |
QuestionMultipleChoice |
Question(‘multiple_choice’, question_name=”””how_feeling”””, question_text=”””How are you?”””, question_options=[‘Good’, ‘Great’, ‘OK’, ‘Bad’], include_comment=False) |
numerical |
QuestionNumerical |
Question(‘numerical’, question_name=”””age”””, question_text=”””You are a 45 year old man. How old are you in years?”””, min_value=0, max_value=86.7, include_comment=False) |
rank |
QuestionRank |
Question(‘rank’, question_name=”””rank_foods”””, question_text=”””Rank your favorite foods.”””, question_options=[‘Pizza’, ‘Pasta’, ‘Salad’, ‘Soup’], num_selections=2) |
top_k |
QuestionTopK |
Question(‘top_k’, question_name=”””two_fruits”””, question_text=”””Which of the following fruits do you prefer?”””, min_selections=2, max_selections=2, question_options=[‘apple’, ‘banana’, ‘carrot’, ‘durian’], use_code=True) |
yes_no |
QuestionYesNo |
Question(‘yes_no’, question_name=”””is_it_equal”””, question_text=”””Is 5 + 5 equal to 11?”””, question_options=[‘No’, ‘Yes’]) |
We can inspect the components of a particular question type by importing the question type class and calling the example method on it:
from edsl import (
# QuestionCheckBox,
# QuestionExtract,
# QuestionFreeText,
# QuestionFunctional,
# QuestionLikertFive,
# QuestionLinearScale,
# QuestionList,
QuestionMultipleChoice,
# QuestionNumerical,
# QuestionRank,
# QuestionTopK,
# QuestionYesNo
)
q = QuestionMultipleChoice.example() # substitute any question type class name
q
Output:
key |
value |
---|---|
question_name |
how_feeling |
question_text |
How are you? |
question_options:0 |
Good |
question_options:1 |
Great |
question_options:2 |
OK |
question_options:3 |
Bad |
include_comment |
False |
question_type |
multiple_choice |
Here we create a simple multiple choice question:
from edsl import QuestionMultipleChoice
q = QuestionMultipleChoice(
question_name = "smallest_prime",
question_text = "Which is the smallest prime number?",
question_options = [0, 1, 2, 3]
)
We can administer it to a language model by calling the run() method on it.
results = q.run()
This generates a dataset of Results that we can readily access with built-in methods for analysis. Here we inspect the response, together with the model that was used and the model’s “comment” about its response–a field that is automatically added to all question types other than free text:
results.select("model", "smallest_prime", "smallest_prime_comment")
Output:
model.model |
answer.smallest_prime |
comment.smallest_prime_comment |
---|---|---|
gpt-4o |
2 |
2 is the smallest prime number because it is the only even number greater than 1 that is divisible only by 1 and itself. |
The Results also include information about the question, model parameters, prompts, generated tokens and raw responses. To see a list of all the components:
results.columns
Output:
0 |
---|
agent.agent_instruction |
agent.agent_name |
answer.smallest_prime |
comment.smallest_prime_comment |
generated_tokens.smallest_prime_generated_tokens |
iteration.iteration |
model.frequency_penalty |
model.logprobs |
model.max_tokens |
model.model |
model.presence_penalty |
model.temperature |
model.top_logprobs |
model.top_p |
prompt.smallest_prime_system_prompt |
prompt.smallest_prime_user_prompt |
question_options.smallest_prime_question_options |
question_text.smallest_prime_question_text |
question_type.smallest_prime_question_type |
raw_model_response.smallest_prime_cost |
raw_model_response.smallest_prime_one_usd_buys |
raw_model_response.smallest_prime_raw_model_response |
Note: If we are running the job locally we can pass run(progress_bar=True) to view a Progress Report. Any exceptions will appear in the console.
If remote inference is activated, a link to a Progress Report will appear automatically, as well as a link to an Exceptions Report if there are any. When the job is completed, a link to the Results page will also appear.
Example: Conducting a survey with agents and models
In the next example we construct a more complex survey consisting of multiple questions and design personas for AI agents to answer it. Then we select specific language models to generate the answers.
We start by creating questions in different types and passing them to a Survey:
from edsl import QuestionLinearScale, QuestionFreeText
q_enjoy = QuestionLinearScale(
question_name = "enjoy",
question_text = "On a scale from 1 to 5, how much do you enjoy reading?",
question_options = [1, 2, 3, 4, 5],
option_labels = {1:"Not at all", 5:"Very much"}
)
q_favorite_place = QuestionFreeText(
question_name = "favorite_place",
question_text = "Describe your favorite place for reading."
)
We construct a Survey by passing a list of questions:
from edsl import Survey
survey = Survey(questions = [q_enjoy, q_favorite_place])
Agents
An important feature of EDSL is the ability to create AI agents to answer questions. This is done by passing dictionaries of relevant “traits” to Agent objects that are used by language models to generate responses. Learn more about designing agents.
Here we construct several simple agent personas to use with our survey:
from edsl import AgentList, Agent
agents = AgentList(
Agent(traits = {"persona":p}) for p in ["artist", "mechanic", "sailor"]
)
Language models
EDSL works with many popular large language models that we can select to use with a survey. This makes it easy to compare responses among models in the results that are generated.
To see a current list of available models:
from edsl import Model
# Model.available() # uncomment this code and run it to see the list of available models
To check the default model that will be used if no models are specified for a survey (e.g., as in the first example above):
Model()
Output (may be different if the default model has changed):
key |
value |
---|---|
model |
gpt-4o |
parameters:temperature |
0.5 |
parameters:max_tokens |
1000 |
parameters:top_p |
1 |
parameters:frequency_penalty |
0 |
parameters:presence_penalty |
0 |
parameters:logprobs |
False |
parameters:top_logprobs |
3 |
Here we select some models to use with our survey:
from edsl import ModelList, Model
models = ModelList(
Model(m) for m in ["gpt-4o", "gemini-pro"]
)
Running a survey
We add agents and models to a survey using the by method. Then we administer a survey the same way that we do an individual question, by calling the run method on it:
results = survey.by(agents).by(models).run()
(
results
.sort_by("persona", "model")
.select("model", "persona", "enjoy", "favorite_place")
)
Example output:
model.model |
agent.persona |
answer.enjoy |
answer.favorite_place |
---|---|---|---|
gemini-pro |
artist |
5 |
Nestled amidst the verdant embrace of a sprawling park, my favorite reading sanctuary unfolds as a secluded haven where tranquility reigns supreme. Beneath the towering canopy of ancient oak trees, a quaint bench beckons, its weathered surface inviting me to sink into its embrace. As I settle in, the gentle rustling of leaves overhead creates a soothing symphony that calms my mind and prepares me for the literary journey ahead. The air is fragrant with the sweet scent of blooming wildflowers, carried by a soft breeze that whispers secrets through the trees. The vibrant hues of nature paint the canvas around me, inspiring a sense of wonder and connection to the world. As I open the pages of my chosen book, the outside world fades into oblivion. The words dance before my eyes, inviting me into realms unknown. The characters become my companions, their stories unfolding before me like a captivating tapestry. Time seems to stand still in this idyllic setting. The worries of the day dissolve as I immerse myself in the written word. As the sun begins its descent, casting long shadows across the park, I close my book and savor the lingering glow of the day. The world around me has transformed into a magical realm, where the boundaries between reality and imagination blur. |
gpt-4o |
artist |
4 |
My favorite place for reading is a cozy nook by a large window in my art studio. The natural light that streams in during the day is perfect for both reading and painting. I have a comfortable armchair draped with a colorful throw, and a small wooden side table where I keep a steaming cup of herbal tea. The walls are adorned with my paintings, which add a touch of inspiration and creativity to the atmosphere. It’s a quiet, peaceful space where I can lose myself in a good book or simply gaze out at the changing scenery outside. |
gemini-pro |
mechanic |
5 |
In the heart of my cozy abode, where solitude and inspiration intertwine, lies my sanctuary of literary bliss—my reading nook. Bathed in the warm glow of a vintage lamp, it beckons me with its allure, a haven where I can escape into the realms of imagination. The walls are adorned with shelves brimming with an eclectic collection of books, their spines whispering tales of adventure, romance, and wisdom. The air is infused with the faint scent of paper and ink, a symphony that awakens my senses. A plush armchair, upholstered in soft velvet, invites me to sink into its embrace, enveloping me in a cocoon of comfort. A large window frames the verdant garden outside, offering a tranquil view of nature’s artistry. As I turn the pages, the rustling of leaves and the chirping of birds create a soothing soundtrack that enhances my reading experience. The gentle breeze carries the sweet fragrance of blooming flowers, mingling with the scent of freshly brewed coffee on my side table. In this tranquil haven, I am free to lose myself in the written word. Time seems to stand still as I journey through distant lands, unravel mysteries, and explore the depths of human emotion. The characters become my companions, their struggles and triumphs mirroring my own. |
gpt-4o |
mechanic |
2 |
As a mechanic, my favorite place for reading might not be what you’d expect. I enjoy reading in my garage, surrounded by the hum of engines and the smell of oil. There’s something comforting about being in my element, with tools and parts all around me. I usually set up a small corner with a sturdy chair and a good lamp, so I can dive into a book during my breaks. Whether it’s a manual on the latest automotive technology or a novel to unwind, the garage is my go-to spot. |
gemini-pro |
sailor |
5 |
Amidst the bustling city’s cacophony, I seek solace in a sanctuary of tranquility—my favorite reading nook. Nestled in a cozy corner of my apartment, it is an oasis of serenity. The soft glow of a vintage lamp illuminates a comfortable armchair, its plush cushions inviting me to sink into its embrace. A large window frames a vibrant cityscape, providing a backdrop of constant movement and life. Yet, within this cozy haven, I find stillness and escape. The walls are adorned with an eclectic collection of artwork, each piece evoking a different memory or inspiration. A vibrant abstract painting captures the essence of a stormy sea, while a delicate watercolor depicts the serene beauty of a mountain meadow. These visual cues transport me to distant realms, setting the stage for literary adventures. The air is scented with the faint aroma of freshly brewed coffee and the subtle fragrance of old books. The gentle hum of the city outside fades into a distant murmur, creating an atmosphere conducive to deep contemplation and immersion. As I settle into my armchair, I reach for a book. Its pages hold the promise of countless worlds to explore, characters to meet, and lessons to learn. The weight of the book in my hands feels both comforting and exhilarating, a tangible connection to the boundless possibilities within its covers. With each turn of the page, I am transported to different times and places. I witness the rise and fall of empires, the triumphs and tragedies of human lives, and the wonders of the natural world. The words dance before my eyes, painting vivid images in my mind. I become lost in the stories, my own worries and concerns fading away. |
gpt-4o |
sailor |
3 |
Ah, my favorite place for reading has to be the deck of a ship, with the vast ocean stretching out endlessly before me. There’s something about the gentle rocking of the waves and the salty sea breeze that makes any book come alive. I love settling into a sturdy deck chair, perhaps with a mug of strong coffee or a tot of rum by my side, and losing myself in a tale while the sun sets on the horizon, painting the sky with colors that even the best of stories can’t quite capture. The sound of the water lapping against the hull provides a soothing background, making it the perfect spot to dive into a good book. |
Running a survey in the background
If remote inference is activated, we can optionally run the survey in the background and continue working (or not) while waiting for results to be generated:
results = survey.by(agents).by(models).run(background=True)
This will return a link to the progress bar page (as usual), which you can check at any time. You can also check the status of the job by running:
results.fetch()
This will return either a status update or the results. Once the job is completed, you can call the results as usual, e.g.:
results.columns # to view a list of all columns
results.select("answer.*") # to view all answers
Example: Adding context to questions
EDSL provides a variety of ways to add data or content to survey questions. These methods include:
Piping answers to questions into follow-on questions
Adding “memory” of prior questions and answers in a survey when presenting other questions to a model
Parameterizing questions with data, e.g., content from PDFs, CSVs, docs, images or other sources that you want to add to questions
Piping question answers
Here we demonstrate how to pipe the answer to a question into the text of another question. This is done by using a placeholder {{ <question_name>.answer }} in the text of the follow-on question where the answer to the prior question is to be inserted when the survey is run. This causes the questions to be administered in the required order (survey questions are administered asynchronously by default). Learn more about piping question answers.
Here we insert the answer to a numerical question into the text of a follow-on yes/no question:
from edsl import QuestionNumerical, QuestionYesNo, Survey
q1 = QuestionNumerical(
question_name = "random_number",
question_text = "Pick a random number between 1 and 1,000."
)
q2 = QuestionYesNo(
question_name = "prime",
question_text = "Is this a prime number: {{ random_number.answer }}"
)
survey = Survey([q1, q2])
results = survey.run()
We can check the user_prompt for the prime question to verify that that the answer to the random_number question was piped into it:
results.select("random_number", "prime_user_prompt", "prime", "prime_comment")
Example output:
answer.random_number |
prompt.prime_user_prompt |
answer.prime |
comment.prime_comment |
---|---|---|---|
487 |
Is this a prime number: 487 No Yes Only 1 option may be selected. Please respond with just your answer. After the answer, you can put a comment explaining your response. |
No |
487 is not a prime number because it can be divided evenly by 1, 487, and also by 19 and 25. |
Adding “memory” of questions and answers
Here we instead add a “memory” of the first question and answer to the context of the second question. This is done by calling a memory rule and identifying the question(s) to add. Instead of just the answer, information about the full question and answer are presented with the follow-on question text, and no placeholder is used. Learn more about question memory rules.
Here we demonstrate the add_targeted_memory method (we could also use set_full_memory_mode or other memory rules):
from edsl import QuestionNumerical, QuestionYesNo, Survey
q1 = QuestionNumerical(
question_name = "random_number",
question_text = "Pick a random number between 1 and 1,000."
)
q2 = QuestionYesNo(
question_name = "prime",
question_text = "Is the number you picked a prime number?"
)
survey = Survey([q1, q2]).add_targeted_memory(q2, q1)
results = survey.run()
We can again use the user_prompt to verify the context that was added to the follow-on question:
results.select("random_number", "prime_user_prompt", "prime", "prime_comment").table().long()
Example output:
row |
key |
value |
---|---|---|
0 |
answer.random_number |
487 |
0 |
prompt.prime_user_prompt |
Is the number you picked a prime number? No Yes |
Scenarios
We can also add external data or content to survey questions. This can be useful when you want to efficiently create and administer multiple versions of questions at once, e.g., for conducting data labeling tasks. This is done by creating Scenario dictionaries for the data or content to be used with a survey, where the keys match {{ placeholder }} names used in question texts (or question options) and the values are the content to be added. Scenarios can also be used to add metadata to survey results, e.g., data sources or other information that you may want to include in the results for reference but not necessarily include in question texts.
In the next example we revise the prior survey questions about reading to take a parameter for other activities that we may want to add to the questions, and create simple scenarios for some activities. EDSL provides methods for automatically generating scenarios from a variety of data sources, including PDFs, CSVs, docs, images, tables and dicts. We use the from_list method to convert a list of activities into scenarios.
Then we demonstrate how to use scenarios to create multiple versions of our questions either (i) when constructing a survey or (ii) when running it:
In the latter case, the by method is used to add scenarios to a survey of questions with placeholders at the time that it is run (the same way that agents and models are added to a survey). This adds a scenario column to the results with a row for each answer to each question for each scenario.
In the former case, the loop method is used to create a list of versions of a question with the scenarios already added to it; when the questions are passed to a survey and it is run, the results include columns for each individual question; there is no scenario column and a single row for each agent’s answers to all the questions.
Learn more about using scenarios.
Here we create simple scenarios for a list of activities:
from edsl import ScenarioList, Scenario
scenarios = ScenarioList.from_list("activity", ["reading", "running", "relaxing"])
Adding scenarios using the by method
Here we add the scenarios to the survey when we run it, together with any desired agents and models:
from edsl import QuestionLinearScale, QuestionFreeText, Survey
q_enjoy = QuestionLinearScale(
question_name = "enjoy",
question_text = "On a scale from 1 to 5, how much do you enjoy {{ activity }}?",
question_options = [1, 2, 3, 4, 5],
option_labels = {1:"Not at all", 5:"Very much"}
)
q_favorite_place = QuestionFreeText(
question_name = "favorite_place",
question_text = "In a brief sentence, describe your favorite place for {{ activity }}."
)
survey = Survey([q_enjoy, q_favorite_place])
results = survey.by(scenarios).by(agents).by(models).run()
(
results
.filter("model.model == 'gpt-4o'")
.sort_by("activity", "persona")
.select("activity", "persona", "enjoy", "favorite_place")
)
Output:
scenario.activity |
agent.persona |
answer.enjoy |
answer.favorite_place |
---|---|---|---|
reading |
artist |
4 |
My favorite place for reading is a cozy nook by a large window, where the natural light spills over the pages, surrounded by plants and the gentle hum of city life outside. |
reading |
mechanic |
2 |
My favorite place for reading is in my garage, surrounded by the hum of engines and the scent of motor oil, where I can escape into a good book during breaks. |
reading |
sailor |
3 |
Ah, my favorite place for reading is out on the deck of a ship, with the salty sea breeze in my hair and the gentle rocking of the waves beneath me. |
relaxing |
artist |
4 |
My favorite place for relaxing is a sun-dappled studio filled with the scent of fresh paint and the gentle hum of creativity. |
relaxing |
mechanic |
3 |
My favorite place for relaxing is in my garage, tinkering with an old engine, where the hum of tools and the smell of grease help me unwind. |
relaxing |
sailor |
3 |
There’s nothing quite like the gentle sway of a hammock on the deck of a ship, with the sound of the ocean waves lapping against the hull and the salty breeze in the air. |
running |
artist |
2 |
My favorite place for running is a winding forest trail where the sunlight filters through the leaves, creating a dappled pattern on the ground. |
running |
mechanic |
1 |
My favorite place for running is a quiet trail through the woods, where the fresh air and natural surroundings make each step feel refreshing. |
running |
sailor |
2 |
Ah, my favorite place for running is along the rugged coastline, where the salty sea breeze fills the air and the waves crash against the rocks, reminding me of the vastness of the ocean. |
Adding scenarios using the loop method
Here we add scenarios to questions when constructing a survey, as opposed to when running it. When we run the survey the results will include columns for each question and no scenario field. Note that we can also optionally use the scenario key in the question names (they are otherwise incremented by default):
from edsl import QuestionLinearScale, QuestionFreeText
q_enjoy = QuestionLinearScale(
question_name = "enjoy_{{ activity }}", # optional use of scenario key
question_text = "On a scale from 1 to 5, how much do you enjoy {{ activity }}?",
question_options = [1, 2, 3, 4, 5],
option_labels = {1:"Not at all", 5:"Very much"}
)
q_favorite_place = QuestionFreeText(
question_name = "favorite_place_{{ activity }}", # optional use of scenario key
question_text = "In a brief sentence, describe your favorite place for {{ activity }}."
)
Looping the scenarios to create a lists of versions of the enjoy question:
enjoy_questions = q_enjoy.loop(scenarios)
enjoy_questions
Output:
Looping the scenarios to create a lists of versions of the favorite_place question:
favorite_place_questions = q_favorite_place.loop(scenarios)
favorite_place_questions
Output:
[Question('free_text', question_name = """favorite_place_reading""", question_text = """In a brief sentence, describe your favorite place for reading."""),
Question('free_text', question_name = """favorite_place_running""", question_text = """In a brief sentence, describe your favorite place for running."""),
Question('free_text', question_name = """favorite_place_relaxing""", question_text = """In a brief sentence, describe your favorite place for relaxing.""")]
Combining the questions into a survey and running it:
survey = Survey(questions = enjoy_questions + favorite_place_questions)
results = survey.by(agents).by(models).run()
We can see that there are additional question fields and no scenario fields:
results.columns
Output:
0 |
---|
Fields |
agent.agent_instruction |
agent.agent_name |
agent.persona |
answer.enjoy_reading |
answer.enjoy_relaxing |
answer.enjoy_running |
answer.favorite_place_reading |
answer.favorite_place_relaxing |
answer.favorite_place_running |
comment.enjoy_reading_comment |
comment.enjoy_relaxing_comment |
comment.enjoy_running_comment |
comment.favorite_place_reading_comment |
comment.favorite_place_relaxing_comment |
comment.favorite_place_running_comment |
generated_tokens.enjoy_reading_generated_tokens |
generated_tokens.enjoy_relaxing_generated_tokens |
generated_tokens.enjoy_running_generated_tokens |
generated_tokens.favorite_place_reading_generated_tokens |
generated_tokens.favorite_place_relaxing_generated_tokens |
generated_tokens.favorite_place_running_generated_tokens |
iteration.iteration |
model.frequency_penalty |
model.logprobs |
model.maxOutputTokens |
model.max_tokens |
model.model |
model.presence_penalty |
model.stopSequences |
model.temperature |
model.topK |
model.topP |
model.top_logprobs |
model.top_p |
prompt.enjoy_reading_system_prompt |
prompt.enjoy_reading_user_prompt |
prompt.enjoy_relaxing_system_prompt |
prompt.enjoy_relaxing_user_prompt |
prompt.enjoy_running_system_prompt |
prompt.enjoy_running_user_prompt |
prompt.favorite_place_reading_system_prompt |
prompt.favorite_place_reading_user_prompt |
prompt.favorite_place_relaxing_system_prompt |
prompt.favorite_place_relaxing_user_prompt |
prompt.favorite_place_running_system_prompt |
prompt.favorite_place_running_user_prompt |
question_options.enjoy_reading_question_options |
question_options.enjoy_relaxing_question_options |
question_options.enjoy_running_question_options |
question_options.favorite_place_reading_question_options |
question_options.favorite_place_relaxing_question_options |
question_options.favorite_place_running_question_options |
question_text.enjoy_reading_question_text |
question_text.enjoy_relaxing_question_text |
question_text.enjoy_running_question_text |
question_text.favorite_place_reading_question_text |
question_text.favorite_place_relaxing_question_text |
question_text.favorite_place_running_question_text |
question_type.enjoy_reading_question_type |
question_type.enjoy_relaxing_question_type |
question_type.enjoy_running_question_type |
question_type.favorite_place_reading_question_type |
question_type.favorite_place_relaxing_question_type |
question_type.favorite_place_running_question_type |
raw_model_response.enjoy_reading_cost |
raw_model_response.enjoy_reading_one_usd_buys |
raw_model_response.enjoy_reading_raw_model_response |
raw_model_response.enjoy_relaxing_cost |
raw_model_response.enjoy_relaxing_one_usd_buys |
raw_model_response.enjoy_relaxing_raw_model_response |
raw_model_response.enjoy_running_cost |
raw_model_response.enjoy_running_one_usd_buys |
raw_model_response.enjoy_running_raw_model_response |
raw_model_response.favorite_place_reading_cost |
raw_model_response.favorite_place_reading_one_usd_buys |
raw_model_response.favorite_place_reading_raw_model_response |
raw_model_response.favorite_place_relaxing_cost |
raw_model_response.favorite_place_relaxing_one_usd_buys |
raw_model_response.favorite_place_relaxing_raw_model_response |
raw_model_response.favorite_place_running_cost |
raw_model_response.favorite_place_running_one_usd_buys |
raw_model_response.favorite_place_running_raw_model_response |
Here we inspect a subset of results:
(
results
.filter("model.model == 'gpt-4o'")
.sort_by("persona")
.select("persona", "enjoy_reading", "enjoy_running", "enjoy_relaxing", "favorite_place_reading", "favorite_place_running", "favorite_place_relaxing")
)
Output:
agent.persona |
answer.enjoy_reading |
answer.enjoy_running |
answer.enjoy_relaxing |
answer.favorite_place_reading |
answer.favorite_place_running |
answer.favorite_place_relaxing |
artist |
4 |
2 |
4 |
My favorite place for reading is a cozy nook by a large window, where the natural light spills over the pages, surrounded by plants and the gentle hum of city life outside. |
My favorite place for running is a winding forest trail where the sunlight filters through the leaves, creating a dappled pattern on the ground. |
My favorite place for relaxing is a sun-dappled studio filled with the scent of fresh paint and the gentle hum of creativity. |
mechanic |
2 |
1 |
3 |
My favorite place for reading is in my garage, surrounded by the hum of engines and the scent of motor oil, where I can escape into a good book during breaks. |
My favorite place for running is a quiet trail through the woods, where the fresh air and natural surroundings make each step feel refreshing. |
My favorite place for relaxing is in my garage, tinkering with an old engine, where the hum of tools and the smell of grease help me unwind. |
sailor |
3 |
2 |
3 |
Ah, my favorite place for reading is out on the deck of a ship, with the salty sea breeze in my hair and the gentle rocking of the waves beneath me. |
Ah, my favorite place for running is along the rugged coastline, where the salty sea breeze fills the air and the waves crash against the rocks, reminding me of the vastness of the ocean. |
There’s nothing quite like the gentle sway of a hammock on the deck of a ship, with the sound of the ocean waves lapping against the hull and the salty breeze in the air. |
Exploring Results
EDSL comes with built-in methods for analyzing and visualizing survey results. For example, you can call the to_pandas method to convert results into a dataframe:
df = results.to_pandas(remove_prefix=True)
# df # uncomment to view output
The Results object also supports SQL-like queries with the the sql method:
results.sql("""
select model, persona, enjoy_reading, favorite_place_reading
from self
order by 1,2,3
""")
Output:
Posting to the Coop
The Coop is a platform for creating, storing and sharing LLM-based research. It is fully integrated with EDSL and accessible from your workspace or Coop account page. Learn more about creating an account and using the Coop.
We can post any EDSL object to the Coop by call the push method on it, optionally passing a description and visibility status:
results.push(description = "Starter tutorial sample survey results", visibility="public")
Example output (UUIDs will be unique to objects):
{'description': 'Starter tutorial sample survey results',
'object_type': 'results',
'url': 'https://www.expectedparrot.com/content/4ec94be1-2a1a-42bb-a463-9f171341ac30',
'uuid': '4ec94be1-2a1a-42bb-a463-9f171341ac30',
'version': '0.1.38.dev1',
'visibility': 'public'}
To post a notebook:
from edsl import Notebook
notebook = Notebook(path="filename.ipynb")
notebook.push(description="Starter Tutorial", visibility="public")
You can view and download a notebook for this tutorial at the Coop.